We are studying tumor heterogeneity and treatment resistance in numerous clinical trials with extensive imaging endpoints for different types of interventions, such as radiation therapy, chemotherapy and molecular targeted therapies (e.g., VEGFR TKI, AR-directed therapies).
- Pharmacodynamics of Anti-Angiogenic Therapies
- Response Heterogeneity in Metastatic Cancer
- Biologically Conformal Radiation Therapy
- Treatment Response in Acute Myeloid Leukemia
- Melanoma
- Lymphoma
- Therapy that blocks the process by which tumors develop vascular structure
- Assessment of the proliferative and vasculature “flare” during drug holiday using FLT PET/CT
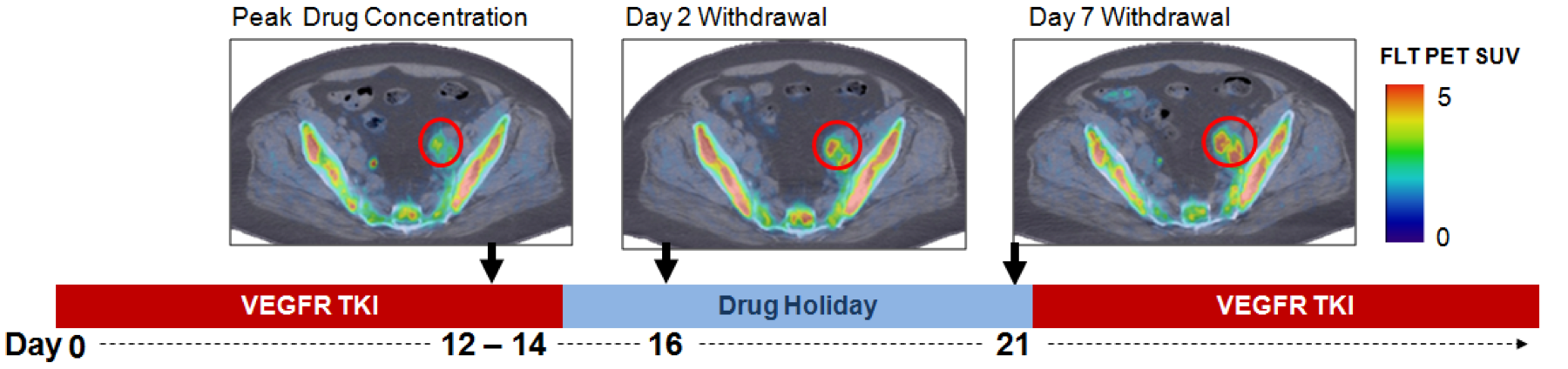
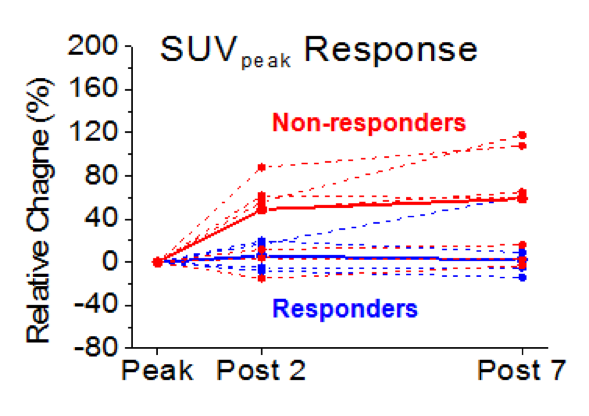
- Assess changes in tumor proliferation and vascular status using static and dynamic FLT PET imaging
- Evaluate imaging biomarkers’ prognostic value for patient outcome (Responders vs. Non-responders
- Future: Extend analysis to later in the treatment cycle (Cycle 3) to probe development of treatment resistance
- Cancer often metastasizes (e.g., mCRPC), which are treated with multiple lines of therapies
- Assessment of total disease burden and disease heterogeneity to assist in drug development and clinical decision making
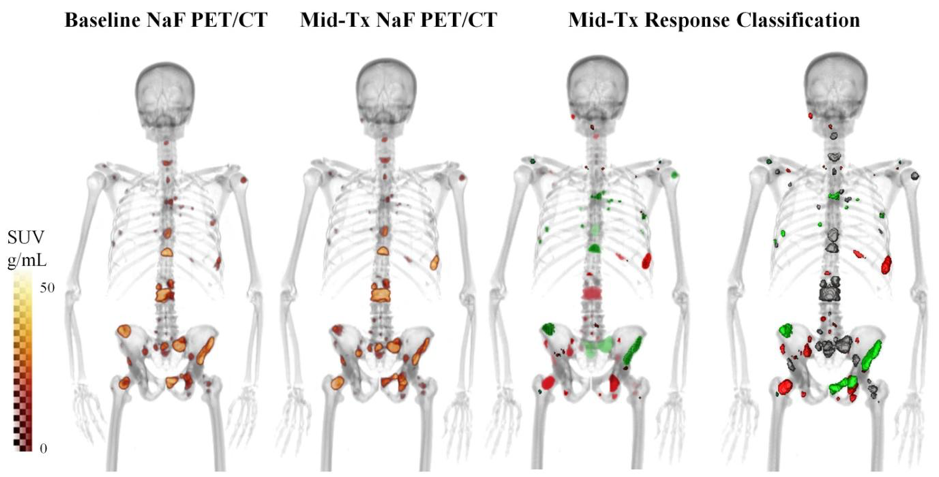
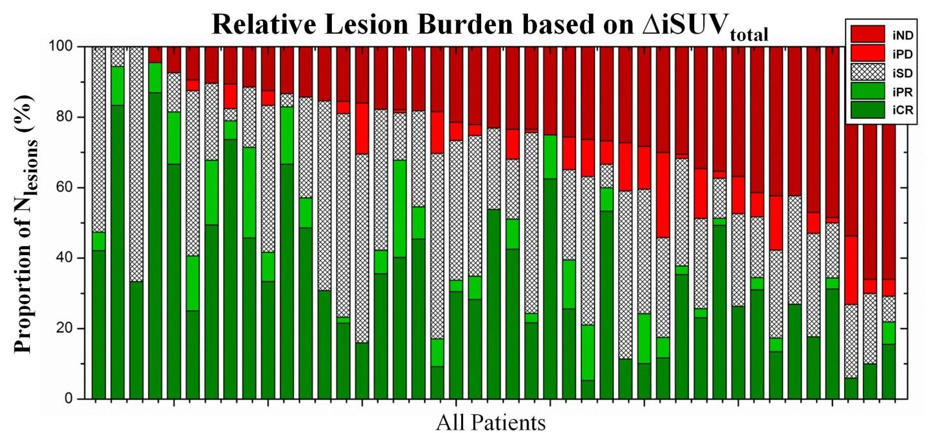
- Strong heterogeneity between responding and non-responding lesions, and dependence on the assessed imaging measure
- Future: Evaluation of the emergence of resistance to multiple therapies
- Dose painting: deliver non-uniform radiation dose to tumors according to spatially-varying radioresistance to increase tumor control probability
- Response in canine sinonasal tumors using extensive molecular imaging
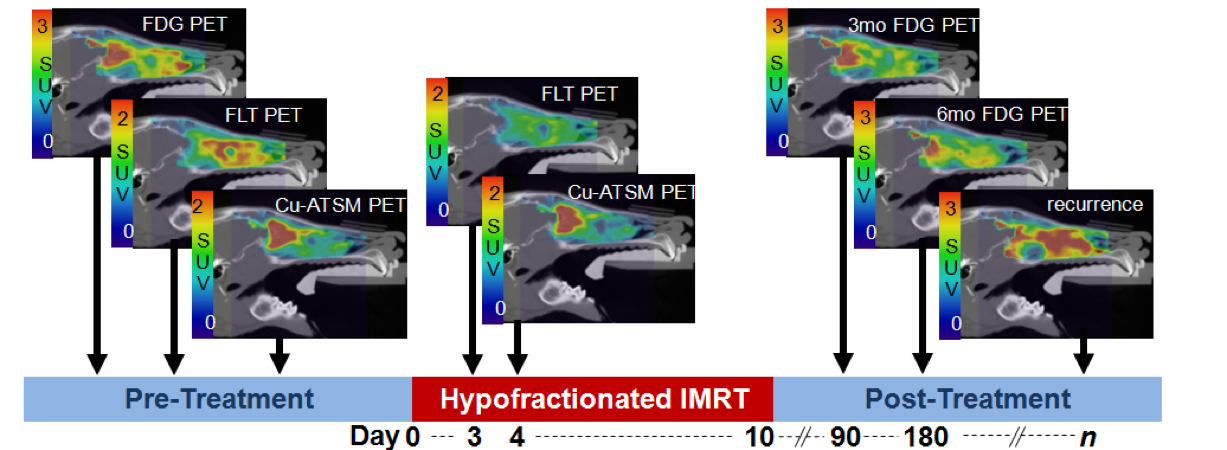
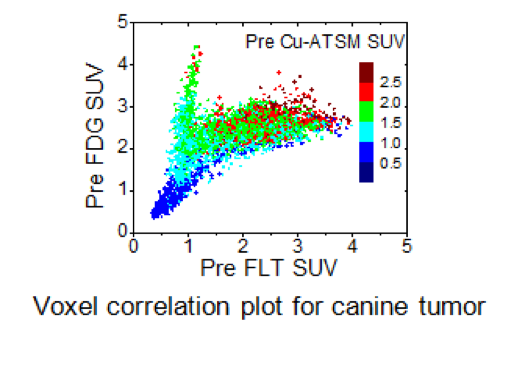
- Strong spatial heterogeneity of radioresistance based on using multi-modality multi-tracer PET imaging
- Determine optimal dose painting method
- Future: Dose painting in human patients
- The current clinical standard for predicting response in AML (Day 14 bone marrow biopsy) has a very poor predictive value (NPV=64%)
- Evaluation of early treatment response assessment using FLT PET/CT as a biomarker
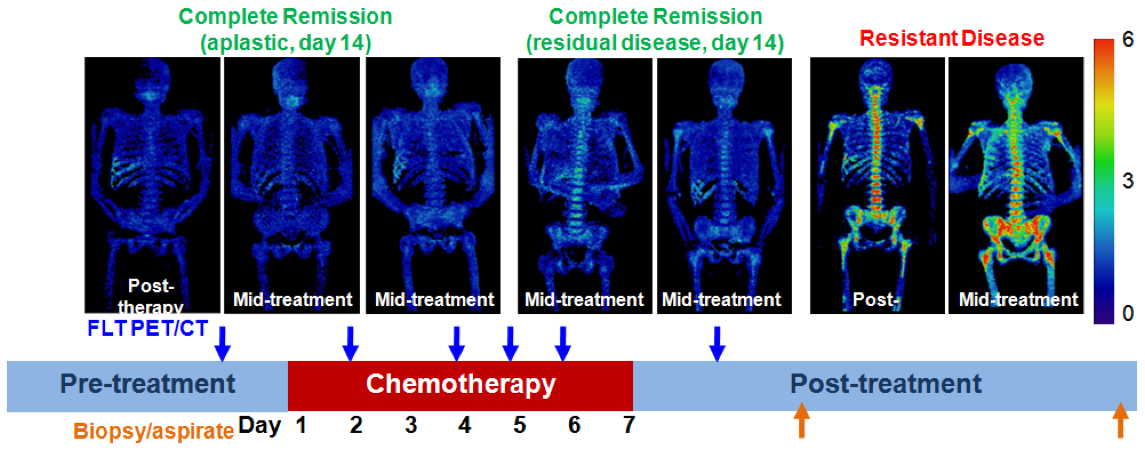
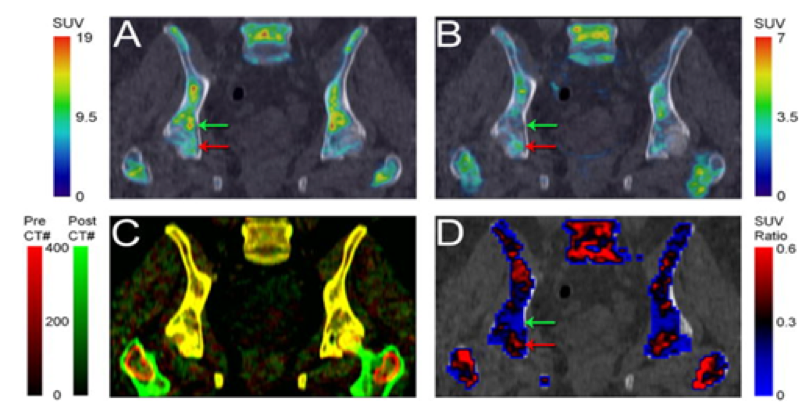
- Assessment of the total bone marrow through FLT PET/CT was shown to be excellent predictor of response
- Multi-center clinical trial through ECOG-ACRIN in preparation
- Future: Assessment of bone marrow and treatment response heterogeneity as a predictive biomarker
- Radiomics is a technique to extract high-dimensional quantitative data from imaging ROIs
- Machine learning classifiers can be trained to predict lesion outcomes from radiomics feature signatures
- Research focus: predicting lesion-level response to immunotherapy in metastatic melanoma
Figure: workflow for lesion response prediction. Left: quantitative features describing lesion uptake, shape, and heterogeneity are extracted from patient imaging, and labelled with a response category. Middle: feature values, represented as vectors in a high dimensional feature space are paired with their corresponding label to serve as training data. Right: classification and regression models are trained on the labelled data to predict lesion response.
- Lymphoma is a challenging disease to assess, as it impacts organs throughout the body such as lymph nodes, bone marrow, and spleen
- It is thus a time-consuming process for physicians to diagnose and assess how lymphoma patients are responding to therapy
- Our group is currently working on developing tools to automatically quantify and assess patients with lymphoma, for use in assisting physicians and providing a “second opinion” on diagnosis and response assessment
- To develop and validate these tools, we are utilizing techniques such as convolutional neural networks, automatic segmentation methods, and hand-crafted feature extraction (radiomics)
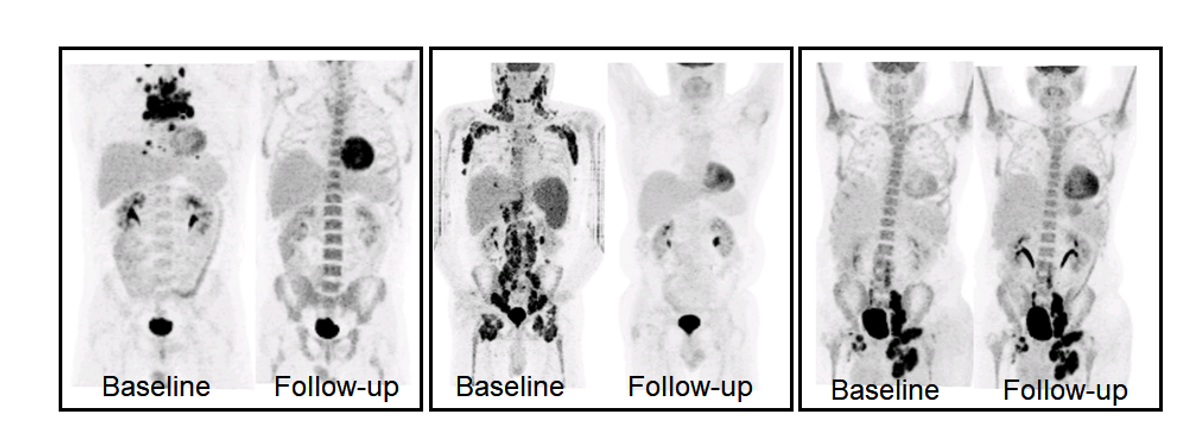